Project Team
The project team consists of a collaboration between members of the University of Waterloo and True North Safety Group. Key project team members from University of Waterloo include:
- Dr. Bruce Hellinga
- Dr. Liping (Lee) Fu
- Dr. Chris Bachmann
- Mohammad Zarei
- Lucas Tito Pereira Sobreira
- Sina Azizisoldouz
and from TNS Group, Dr. Pedram Izadpanah.
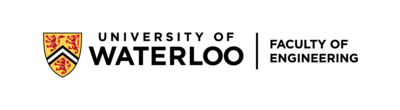
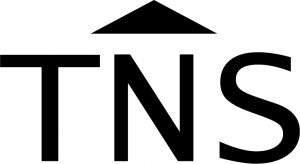
Project Background
Road safety is a critical public health issue in Canada as each year approximately 2,000 people are killed and 165,000 are injured in collisions (CCMTA, 2016[1]). Furthermore, other peer countries show better road safety performance. In 2017, Canada ranked 19th among Organization for Economic Development (OECD) countries based on road fatalities per inhabitant and 10th based on road deaths per distance travelled (ITF, 2018[2]), and Canada remains one of only seven industrialized nations where pedestrian deaths are still increasing – up 10.5% from 2010 to 2016 (ITF, 2018). The annual social costs of the motor vehicle collisions in terms of loss of life, medical treatment, rehabilitation, lost productivity, and property damage are measured in tens of billions of dollars – estimated at $40.7 billion for Canada in 2017 (ITF, 2019[3]).
The Highway Safety Manual (HSM) defines the Roadway Safety Management Process (RSMP) as a six-step approach to improving road safety (HSM, 2010). The first step in the RSMP is Network Screening (NS) which is designed to identify and prioritize the road sites (e.g., intersections, roadway segments, and/or ramps) where design or operation results in an unusually high number of collisions or a higher-than-normal proportion of a certain type of collision, referred to as hotspots. The output of the network screening process is an ordered list of hazardous sites. Road authorities have limited resources and therefore can address only a limited number of sites each year. The objective of network screening is to identify the sites that present the greatest risk and/or have the highest potential for improving safety. When the network screening process is inaccurate then the road authority will allocate resources to the wrong sites, resulting in inefficient use of funds and a failure to achieve the potential safety improvements.
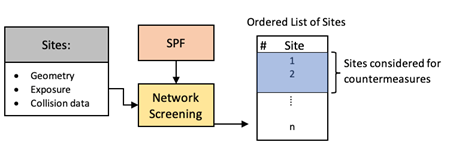
Prevalent network screening methods rely on a set of analytical models known as Safety Performance Functions (SPFs), that are developed on the basis of a database of historical data (i.e. reported collisions and site characteristics such as geometry and exposure). The SPFs are used to predict the number of collisions (by severity) at each site, which is then combined, using an Empirical Bayes (EB) approach, with the observed number of collisions at the site, to obtain the expected number of collisions. SPF-based network screening methods in the HSM include:
- Expected Average Crash Frequency with Empirical Bayes (EB) Adjustment
- Equivalent Property Damage Only (EPDO) Average Crash Frequency with EB Adjustments
- Excess Expected Average Crash Frequency Using SPFs with EB Adjustments
There are four main challenges to carrying out network screening using these methods:
1) Methods that rely solely on historical collisions suffer from limitations such as bias due to low mean and small sample size and regression-to-the-mean (RTM). Furthermore, these methods are reactive, rather than proactive, as they can only identify hazardous sites after collisions have occurred.
2) SPFs must be developed using local data and must be regularly updated (re-developed). This activity is resource intensive, requiring extensive data and specialized statistical and road safety modelling expertise, and therefore posing a barrier for small and medium sized municipalities in Canada. Further work is needed to (i) develop tools to enable municipalities to determine in an objective way if an existing SPF needs to be updated; and (ii) develop methods that permit transferability of an SPF that has been developed in another municipality to a local jurisdiction with minimal recalibration effort.
3) Conventional SPFs consist of analytical forms such as the Negative Binomial model which make assumptions about the distribution of the collision data that are frequently invalid. Emerging AI techniques provide an opportunity to develop models that can better represent collision data and other forms of data that reflect safety.
4) Typically, there is substantial error in the exposure data for motorized vehicles (normally expressed as AADT and estimated from a small number of count stations) and little or no data to quantify exposure of vulnerable road users such as pedestrians and cyclists. This results in both error and bias in the network screening outcomes. Methods are required to improve the accuracy of exposure data for vehicles and VRUs and for NS screening methods that are less sensitive to errors in exposure data.
[1] CCMTA (2016). Canada’s road safety strategy 2025: Towards zero: The safest roads in the world. https://roadsafetystrategy.ca/files/RSS-2025-Report-January-2016-with%20cover.pdf
[2] ITF (2018). Road safety annual report 2018. https://www.itf-oecd.org/sites/default/files/docs/irtad-road-safety-annual-report-2018_2.pdf
[3] ITF (2019). Road safety annual report 2019: Canada. https://www.itf-oecd.org/sites/default/files/canada-road-safety.pdf