5) Network Screening Framework considering Collisions and Safety Surrogate Measures (SMMs)
There are at least two types of measures that can be used to conduct network screening: 1) collision-based measures; and 2) surrogate safety measures (SSMs). In this project, we developed a conceptual framework for networking screening using these measures, either individually or in combination, with varying spatial and road user coverage. The figure below illustrates the structure of the proposed framework.
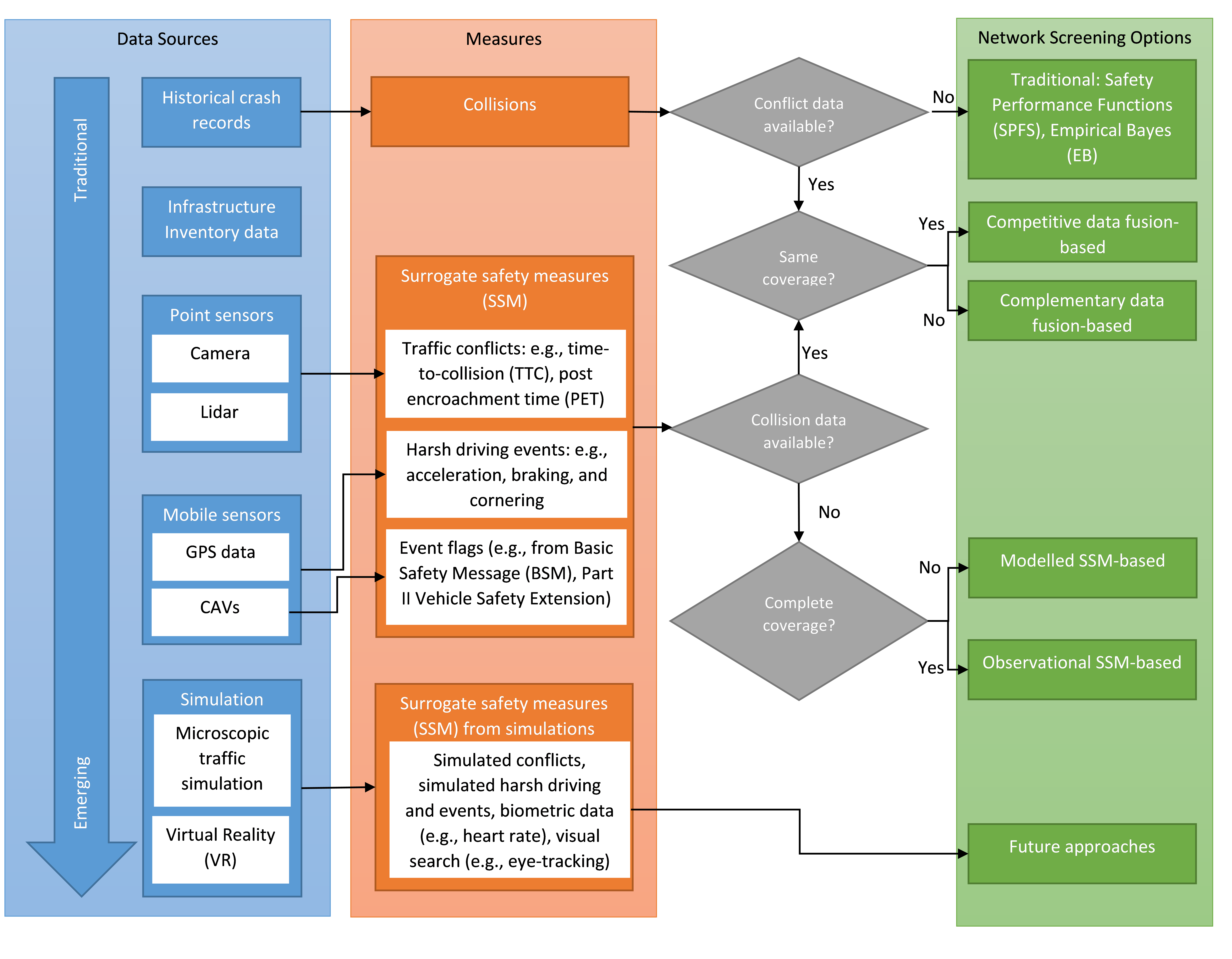
FIGURE: PROPOSED FRAMEWORK FOR NETWORK SCREENING
There are five potential scenarios for conducting network screening:
3.1. Traditional Approach (Collision-based measures)
This option can be used when conflict data are not available for the locations being analyzed. Historical crash data can be used to conduct network screening through naïve or model-based approaches. Naïve methods involve calculating crash rates for each location based on the total number of crashes that occurred in a given period. Model-based methods involve developing Safety Performance Functions (SPFs) using crash data and covariates such as traffic volume and geometric characteristics. The SPFs can then be used in combination with observed crash data (e.g., Empirical Bayes) to predict the number of crashes that are likely to occur at each location.
3.2. Competitive Data Fusion
This option can be selected when conflict data are available for the same spatial and road user type coverage. In this approach, both historical crash data and conflict data collected from point sensors (e.g., cameras, lidar) and/or mobile sensors (e.g., GPS data, CAVs) can be used to conduct safety analysis. One method involves using SSMs as covariates in crash-based SPF estimation. Another method is to separately estimate and predict the crash risk based on historical observed collision and conflict data and then use more sophisticated models such as copula models or meta-analysis models to combine the results from both measures for network screening.
3.3. Complementary Data Fusion
This option assumes that both conflict data and crash data are available, but their coverage is different. For example, crash data may only be available for specific types of road users such as vehicular collisions. In this case, conflict data can be used to estimate the risk of vulnerable road user (VRU) collisions (which are often lacking in observational data), and both conflict-based and collision-based measures can be combined to conduct network screening for all types of road users.
3.4. SSM-based Measures
This alternative can be used when crash data is unavailable, and network screening needs to be conducted based on conflict data. If conflict data is available for all locations, observed conflict measures such as PET and TTC can be used. If conflict data is only available for some locations, conflict indicators can be used to develop models to estimate and predict the number of conflicts for all locations. In this case, all the methods discussed for collision-based measures (i.e., naïve and model-based methods) can be used to conduct safety analysis.
3.5. Simulation-based Analysis
This approach involves collecting data using microtraffic simulation, biometric sensors (e.g., heart rate sensors), virtual reality (VR) technology, and conducting network screening based on these data. This is a potential approach in the future as technology continues to advance.
Limitations/Remaining Questions
Although the proposed framework covers various measures for conducting network screening based on different sources of data, there are several limitations/remaining technical challenges as follows:
- Objectives/purpose of network screening: The framework does not explicitly mention the objectives or purpose of network screening. Depending on the goals, different measures and approaches may be more or less relevant. For example, if the objective is to identify high-risk locations for pedestrians or cyclists, conflict-based measures and complementary data fusion may be more appropriate than other approaches.
- The framework should provide more information about the key questions and thresholds related to each approach. For example, what are the conflict thresholds for identifying high-risk locations? How can we determine the appropriate level of aggregation for network screening (e.g., intersection-level vs mid-block level)? Answering these questions can help to ensure that the measures are applied consistently and accurately across different locations.
- Network elements and scope of network screening: The framework should also consider the network elements and scope of network screening. For example, the effectiveness of conflict-based measures may vary depending on the type of network element (e.g., intersections vs mid-blocks) and the location (urban vs rural, arterials vs freeways). Additionally, the feasibility of using different data sources for network screening may vary depending on the scope of the analysis (e.g., fixed point sensors for conflict analysis for mid-blocks may be less feasible than at signalized intersections).
Addressing these limitations/remaining technical challenges will require more research.
5. References
Aguero-Valverde, J., & Jovanis, P. P. (2008). Analysis of road crash frequency with spatial models. Transportation Research Record, 2061(1), 55-63.
Aguero-Valverde, J., & Jovanis, P. P. (2009). Bayesian multivariate Poisson lognormal models for crash severity modeling and site ranking. Transportation Research Record, 2136(1), 82-91.
Allen, B. L., Shin, B. T., & Cooper, P. J. (1978). Analysis of traffic conflicts and collisions (0361-1981).
Amundsen, F., & Hyden, C. (1977). Proceedings of first workshop on traffic conflicts. Oslo, TTI, Oslo, Norway and LTH Lund, Sweden, 78.
Archer, J. (2005). Indicators for traffic safety assessment and prediction and their application in micro-simulation modelling. Royal Institute of Technology, Stochholm.
Arun, A., Haque, M. M., Bhaskar, A., Washington, S., & Sayed, T. (2021). A systematic mapping review of surrogate safety assessment using traffic conflict techniques. Accident Analysis & Prevention, 153, 106016.
Bachmann, C., Roorda, M. J., & Abdulhai, B. (2011). Improved Time-to-Collision Definition for Simulating Traffic Conflicts on Truck-Only Infrastructure. Transportation research record, 2237(1), 31-40. https://doi.org/10.3141/2237-04
Bernardin, K., & Stiefelhagen, R. (2008). Evaluating multiple object tracking performance: the clear mot metrics. EURASIP Journal on Image and Video Processing, 2008, 1-10.
Bernas, M., Płaczek, B., Korski, W., Loska, P., Smyła, J., & Szymała, P. (2018). A survey and comparison of low-cost sensing technologies for road traffic monitoring. Sensors, 18(10), 3243.
Bhattacharyya, M., & Ritolia, G. (2008). Conditional VaR using EVT–Towards a planned margin scheme. International Review of Financial Analysis, 17(2), 382-395.
Cafiso, S., D’Agostino, C., Kieć, M., & Bak, R. (2018). Safety assessment of passing relief lanes using microsimulation-based conflicts analysis. Accident Analysis & Prevention, 116, 94-102. https://doi.org/https://doi.org/10.1016/j.aap.2017.07.001
Caliendo, C., Guida, M., & Parisi, A. (2007). A crash-prediction model for multilane roads. Accident Analysis & Prevention, 39(4), 657-670.
Cameron, A. C., & Trivedi, P. K. (2013). Regression analysis of count data (Vol. 53). Cambridge university press.
Campbell, K. L. (1996). A bridging analysis for estimating the benefits of active safety technologies.
Canada, T. (2020). Canadian Motor Vehicle Traffic Collision Statistics: 2020. https://tc.canada.ca/en/road-transportation/statistics-data/canadian-motor-vehicle-traffic-collision-statistics-2020
Casella, G., & Berger, R. (2001). Hypothesis testing in statistics.
Cheng, L., Geedipally, S. R., & Lord, D. (2013). The Poisson–Weibull generalized linear model for analyzing motor vehicle crash data. Safety science, 54, 38-42.
Cheng, W., Gill, G. S., Ensch, J. L., Kwong, J., & Jia, X. (2018). Multimodal crash frequency modeling: Multivariate space-time models with alternate spatiotemporal interactions. Accident Analysis & Prevention, 113, 159-170.
Chin, H. C., & Quddus, M. A. (2003). Applying the random effect negative binomial model to examine traffic accident occurrence at signalized intersections. Accident Analysis & Prevention, 35(2), 253-259.
Connors, R. D., Maher, M., Wood, A., Mountain, L., & Ropkins, K. (2013). Methodology for fitting and updating predictive accident models with trend. Accident Analysis & Prevention, 56, 82-94.
Cooper, D. F., & Ferguson, N. (1976). Traffic studies at T-Junctions. 2. A conflict simulation Record. Traffic Engineering & Control, 17(Analytic).
Council, F. M., Reinfurt, D. W., Campbell, B., Roediger, F. L., Carroll, C. L., Dutt, A. K., & Dunham, J. R. (1980). Accident research manual.
Datondji, S. R. E., Dupuis, Y., Subirats, P., & Vasseur, P. (2016). A survey of vision-based traffic monitoring of road intersections. IEEE transactions on intelligent transportation systems, 17(10), 2681-2698.
Davis, G. A., Hourdos, J., Xiong, H., & Chatterjee, I. (2011). Outline for a causal model of traffic conflicts and crashes. Accident Analysis & Prevention, 43(6), 1907-1919. https://doi.org/https://doi.org/10.1016/j.aap.2011.05.001
Dingus, T. A., Klauer, S. G., Neale, V. L., Petersen, A., Lee, S. E., Sudweeks, J., Perez, M. A., Hankey, J., Ramsey, D., & Gupta, S. (2006). The 100-car naturalistic driving study, Phase II-results of the 100-car field experiment.
El-Basyouny, K., & Sayed, T. (2006). Comparison of two negative binomial regression techniques in developing accident prediction models. Transportation Research Record, 1950(1), 9-16.
El-Basyouny, K., & Sayed, T. (2013). Safety performance functions using traffic conflicts. Safety Science, 51(1), 160-164. https://doi.org/https://doi.org/10.1016/j.ssci.2012.04.015
Elvik, R. (2007). State-of-the-art approaches to road accident black spot management and safety analysis of road networks. Transportøkonomisk institutt Oslo.
Ettehadieh, D. (2014). Systematic parameter optimization and application of automated tracking in pedestrian-dominant situations École Polytechnique de Montréal].
Farah, H., & Azevedo, C. L. (2017). Safety analysis of passing maneuvers using extreme value theory. IATSS research, 41(1), 12-21. https://doi.org/https://doi.org/10.1016/j.iatssr.2016.07.001
Formosa, N., Quddus, M., Ison, S., Abdel-Aty, M., & Yuan, J. (2020). Predicting real-time traffic conflicts using deep learning. Accident Analysis & Prevention, 136, 105429. https://doi.org/https://doi.org/10.1016/j.aap.2019.105429
Forsyth, D. A., Arikan, O., Ikemoto, L., O'Brien, J., & Ramanan, D. (2006). Computational studies of human motion: Part 1, tracking and motion synthesis. Foundations and Trends® in Computer Graphics and Vision, 1(2–3), 77-254.
Gelman, A., Carlin, J. B., Stern, H. S., & Rubin, D. B. (1995). Bayesian data analysis. Chapman and Hall/CRC.
Ghanipoor Machiani, S., & Abbas, M. (2016). Safety surrogate histograms (SSH): A novel real-time safety assessment of dilemma zone related conflicts at signalized intersections. Accident Analysis & Prevention, 96, 361-370. https://doi.org/https://doi.org/10.1016/j.aap.2015.04.024
Greene, W. H. (2003). Econometric analysis. Pearson Education India.
Gupta, N., Jashami, H., Savolainen, P. T., Abouali, M., Barrette, T., Coplai, C., & Powell, W. (2021). Connected vehicle data validation: How do CV events relate to collision trends? Michigan State University. https://safetyinsights.ford.com/assets/resources/w4.pdf
Gustavsson, J. (1969). On the use of regression models in the study of road accidents. Accident Analysis & Prevention.
Gustavsson, J., & Svensson, Å. (1976). A Poisson regression model applied to classes of road accidents with small frequencies. Scandinavian Journal of Statistics, 49-60.
Hauer, E. (1982). Traffic conflicts and exposure. Accident Analysis & Prevention, 14(5), 359-364. https://doi.org/https://doi.org/10.1016/0001-4575(82)90014-8
Hauer, E. (1992). Empirical Bayes approach to the estimation of “unsafety”: the multivariate regression method. Accident Analysis & Prevention, 24(5), 457-477.
Hauer, E. (1997). Observational before/after studies in road safety. estimating the effect of highway and traffic engineering measures on road safety.
Hauer, E., & Garder, P. (1986). Research into the validity of the traffic conflicts technique. Accident Analysis & Prevention, 18(6), 471-481. https://doi.org/https://doi.org/10.1016/0001-4575(86)90020-5
Hauer, E., Ng, J. C., & Lovell, J. (1988). Estimation of safety at signalized intersections. Transportation Research Record, 1185, 48-61.
Huang, H., Chin, H. C., & Haque, M. (2008). Bayesian hierarchical analysis on crash prediction models. TRB 87th Annual Meeting Compendium of Papers DVD,
Huang, H., Chin, H. C., & Haque, M. M. (2009). Empirical evaluation of alternative approaches in identifying crash hot spots: Naive ranking, empirical bayes, full bayes methods. Transportation Research Record, 2103(1), 32-41.
Hupfer, C. (1997). Deceleration to safety time (DST)-a useful figure to evaluate traffic safety. ICTCT Conference Proceedings of Seminar,
Ismail, K., Sayed, T., & Saunier, N. (2011). Methodologies for Aggregating Indicators of Traffic Conflict. Transportation research record, 2237(1), 10-19. https://doi.org/10.3141/2237-02
Ismail, K., Sayed, T., Saunier, N., & Lim, C. (2009). Automated analysis of pedestrian–vehicle conflicts using video data. Transportation research record, 2140(1), 44-54.
Jackson, S., Miranda-Moreno, L. F., St-Aubin, P., & Saunier, N. (2013). Flexible, mobile video camera system and open source video analysis software for road safety and behavioral analysis. Transportation research record, 2365(1), 90-98.
Jiang, X., Huang, B., Zaretzki, R. L., Richards, S., & Yan, X. (2013). Estimating safety effects of pavement management factors utilizing Bayesian random effect models. Traffic injury prevention, 14(7), 766-775.
Joshua, S. C., & Garber, N. J. (1990). Estimating truck accident rate and involvements using linear and Poisson regression models. Transportation planning and Technology, 15(1), 41-58.
Jovanis, P. P., & Chang, H.-L. (1986). Modeling the relationship of accidents to miles traveled. Transportation Research Record, 1068, 42-51.
Kamrani, M., Wali, B., & Khattak, A. J. (2017). Can data generated by connected vehicles enhance safety?: Proactive approach to intersection safety management. Transportation research record, 2659(1), 80-90.
Kaparias, I., Bell, M. G. H., Greensted, J., Cheng, S., Miri, A., Taylor, C., & Mount, B. (2010). Development and Implementation of a Vehicle–Pedestrian Conflict Analysis Method: Adaptation of a Vehicle–Vehicle Technique. Transportation research record, 2198(1), 75-82. https://doi.org/10.3141/2198-09
Kim, T., Lieberman, B., Luta, G., & Peña, E. A. (2022). Prediction intervals for Poisson‐based regression models. Wiley Interdisciplinary Reviews: Computational Statistics, 14(5), e1568.
Klebelsberg, D. (1964). Derzeitiger Sand der Verhaltensanalyse des Kraftfahrens, Zrbeit und Leistung. Ablt. Arbeitswissenscaft Soziale Betriebspraxis, 18, 33-37. https://www.scopus.com/inward/record.uri?eid=2-s2.0-84856078522&partnerID=40&md5=7ebe53771c832235f8b77f94cd0d4811
Kononov, J., & Allery, B. (2003). Level of service of safety: Conceptual blueprint and analytical framework. Transportation Research Record, 1840(1), 57-66.
Kononov, J., Durso, C., Lyon, C., & Allery, B. (2015). Level of service of safety revisited. Transportation Research Record, 2514(1), 10-20.
Lai, Z., Karim, I., & Xianghai, M. (2014). Traffic conflict techniques for road safety analysis: open questions and some insights. Canadian Journal of Civil Engineering, 41(7), 633-641. https://doi.org/10.1139/cjce-2013-0558
Lan, B., & Persaud, B. (2012). Evaluation of multivariate Poisson log normal Bayesian methods for before-after road safety evaluations. Journal of Transportation Safety & Security, 4(3), 193-210.
Laughland, J. C., Haefner, L. E., Hall, J. W., & Clough, D. R. (1975). Methods for evaluating highway safety improvements (0077-5614).
Laureshyn, A., Ardö, H., Svensson, Å., & Jonsson, T. (2009). Application of automated video analysis for behavioural studies: concept and experience. IET Intelligent Transport Systems, 3(3), 345-357.
Lawless, J. F. (1987). Negative binomial and mixed Poisson regression. The Canadian Journal of Statistics/La Revue Canadienne de Statistique, 209-225.
Liu, J., & Khattak, A. J. (2016). Delivering improved alerts, warnings, and control assistance using basic safety messages transmitted between connected vehicles. Transportation Research Part C: Emerging Technologies, 68, 83-100.
Lord, D., & Mannering, F. (2010). The statistical analysis of crash-frequency data: A review and assessment of methodological alternatives. Transportation research part A: policy and practice, 44(5), 291-305.
Lord, D., & Miranda-Moreno, L. F. (2008). Effects of low sample mean values and small sample size on the estimation of the fixed dispersion parameter of Poisson-gamma models for modeling motor vehicle crashes: A Bayesian perspective. Safety science, 46(5), 751-770.
Lord, D., & Washington, S. (2018). Safe mobility: Challenges, methodology and solutions. Emerald Group Publishing.
Lord, D., Washington, S. P., & Ivan, J. N. (2005). Poisson, Poisson-gamma and zero-inflated regression models of motor vehicle crashes: balancing statistical fit and theory. Accident Analysis & Prevention, 37(1), 35-46.
MacNab, Y. C. (2003). A Bayesian hierarchical model for accident and injury surveillance. Accident Analysis & Prevention, 35(1), 91-102.
Mannering, F. (2018). Temporal instability and the analysis of highway accident data. Analytic Methods in Accident Research, 17, 1-13.
Mannering, F., Bhat, C. R., Shankar, V., & Abdel-Aty, M. (2020). Big data, traditional data and the tradeoffs between prediction and causality in highway-safety analysis. Analytic Methods in Accident Research, 25, 100113.
Mannering, F. L., & Bhat, C. R. (2014). Analytic methods in accident research: Methodological frontier and future directions. Analytic Methods in Accident Research, 1, 1-22.
Miaou, S.-P. (1994). The relationship between truck accidents and geometric design of road sections: Poisson versus negative binomial regressions. Accident Analysis & Prevention, 26(4), 471-482.
Miaou, S.-P., & Lord, D. (2003). Modeling traffic crash-flow relationships for intersections: dispersion parameter, functional form, and Bayes versus empirical Bayes methods. Transportation Research Record, 1840(1), 31-40.
Miaou, S.-P., & Lum, H. (1993). Modeling vehicle accidents and highway geometric design relationships. Accident Analysis & Prevention, 25(6), 689-709.
Miaou, S.-P., & Song, J. J. (2005). Bayesian ranking of sites for engineering safety improvements: decision parameter, treatability concept, statistical criterion, and spatial dependence. Accident Analysis & Prevention, 37(4), 699-720.
Minderhoud, M. M., & Bovy, P. H. (2001). Extended time-to-collision measures for road traffic safety assessment. Accident Analysis & Prevention, 33(1), 89-97.
Miranda-Moreno, L. F., Fu, L., Saccomanno, F. F., & Labbe, A. (2005). Alternative risk models for ranking locations for safety improvement. Transportation Research Record, 1908(1), 1-8.
Miranda-Moreno, L. F., Heydari, S., Lord, D., & Fu, L. (2013). Bayesian road safety analysis: Incorporation of past evidence and effect of hyper-prior choice. Journal of safety research, 46, 31-40.
Miranda-Moreno, L. F., Labbe, A., & Fu, L. (2007). Bayesian multiple testing procedures for hotspot identification. Accident Analysis & Prevention, 39(6), 1192-1201.
Montella, A. (2010). A comparative analysis of hotspot identification methods. Accident Analysis & Prevention, 42(2), 571-581.
National Research Council, T. R. B. (2010). Highway safety manual (Vol. 1). AASHTO.
Ozbay, K., Yang, H., Bartin, B., & Mudigonda, S. (2008). Derivation and Validation of New Simulation-Based Surrogate Safety Measure. Transportation research record, 2083(1), 105-113. https://doi.org/10.3141/2083-12
Papadoulis, A., Quddus, M., & Imprialou, M. (2019). Evaluating the safety impact of connected and autonomous vehicles on motorways. Accident Analysis & Prevention, 124, 12-22.
Park, E. S., Carlson, P. J., Porter, R. J., & Andersen, C. K. (2012). Safety effects of wider edge lines on rural, two-lane highways. Accident Analysis & Prevention, 48, 317-325.
Park, E. S., & Lord, D. (2007). Multivariate Poisson-lognormal models for jointly modeling crash frequency by severity. Transportation Research Record, 2019(1), 1-6.
Qin, X., Ng, M., & Reyes, P. E. (2010). Identifying crash-prone locations with quantile regression. Accident Analysis & Prevention, 42(6), 1531-1537.
Rigby, R. A., Stasinopoulos, D. M., & Akantziliotou, C. (2008). A framework for modelling overdispersed count data, including the Poisson-shifted generalized inverse Gaussian distribution. Computational Statistics & Data Analysis, 53(2), 381-393.
Salamati, K., Schroeder, B., Rouphail, N. M., Cunningham, C., Long, R., & Barlow, J. (2011). Development and Implementation of Conflict-Based Assessment of Pedestrian Safety to Evaluate Accessibility of Complex Intersections. Transportation research record, 2264(1), 148-155. https://doi.org/10.3141/2264-17
Salman, N. K., & Al-Maita, K. J. (1995). Safety evaluation at three-leg, unsignalized intersections by traffic conflict technique. Transportation research record, 1485(28), 177-185.
Saunier, N., Sayed, T., & Ismail, K. (2010). Large-scale automated analysis of vehicle interactions and collisions. Transportation research record, 2147(1), 42-50.
Savolainen, P. T., Mannering, F. L., Lord, D., & Quddus, M. A. (2011). The statistical analysis of highway crash-injury severities: a review and assessment of methodological alternatives. Accident Analysis & Prevention, 43(5), 1666-1676.
Sayed, T., & Zein, S. (1999). Traffic conflict standards for intersections. Transportation planning and technology, 22(4), 309-323.
Shankar, V. N., Albin, R. B., Milton, J. C., & Mannering, F. L. (1998). Evaluating median crossover likelihoods with clustered accident counts: An empirical inquiry using the random effects negative binomial model. Transportation Research Record, 1635(1), 44-48.
Shanker, V., Milton, J., & Mannering, F. (1997). Modeling accident frequencies as zero-altered probability process. Accident Analysis and Prevention, 29, 829-837.
Songchitruksa, P., & Tarko, A. P. (2006). The extreme value theory approach to safety estimation. Accident Analysis & Prevention, 38(4), 811-822. https://doi.org/https://doi.org/10.1016/j.aap.2006.02.003
Songchitruksa, P., & Zha, L. (2014). Advancing safety performance monitoring at signalized intersections through use of connected vehicle technology. Transportation research record, 2432(1), 99-109.
Srinivasan, R., Baek, J., & Council, F. (2010). Safety evaluation of transverse rumble strips on approaches to stop-controlled intersections in rural areas. Journal of Transportation Safety & Security, 2(3), 261-278.
St-Aubin, P., Saunier, N., & Miranda-Moreno, L. (2015). Large-scale automated proactive road safety analysis using video data. Transportation Research Part C: Emerging Technologies, 58, 363-379.
Stipancic, J., Miranda-Moreno, L., Saunier, N., & Labbe, A. (2018). Surrogate safety and network screening: Modelling crash frequency using GPS travel data and latent Gaussian Spatial Models. Accident Analysis & Prevention, 120, 174-187. https://doi.org/https://doi.org/10.1016/j.aap.2018.07.013
Stylianou, K., & Dimitriou, L. (2018). Analysis of rear-end conflicts in urban networks using Bayesian networks. Transportation research record, 2672(38), 302-312.
Sun, Y., Xu, H., Wu, J., Zheng, J., & Dietrich, K. M. (2018). 3-D data processing to extract vehicle trajectories from roadside LiDAR data. Transportation research record, 2672(45), 14-22.
Tageldin, A., & Sayed, T. (2019). Models to evaluate the severity of pedestrian-vehicle conflicts in five cities. Transportmetrica A Transport Science, 15(2), 354-375. https://doi.org/https://doi.org/10.1080/23249935.2018.1477853
Tarko, A. P. (2012). Use of crash surrogates and exceedance statistics to estimate road safety. Accident Analysis & Prevention, 45, 230-240. https://doi.org/https://doi.org/10.1016/j.aap.2011.07.008
Tarko, A. P. (2018). Estimating the expected number of crashes with traffic conflicts and the Lomax Distribution – A theoretical and numerical exploration. Accident Analysis & Prevention, 113, 63-73. https://doi.org/https://doi.org/10.1016/j.aap.2018.01.008
Tarko, A. P. (2018). Surrogate measures of safety. In Safe mobility: challenges, methodology and solutions. Emerald Publishing Limited.
Tarko, A. P., Ariyur, K. B., Romero, M., Bandaru, V. K., & Jimenez, C. L. (2016). Guaranteed LiDAR-aided multi-object tracking at road intersections: USDOT Region V Regional University Transportation Center final report.
Tian, Y., Liu, H., & Furukawa, T. (2017). Reliable infrastructural urban traffic monitoring via lidar and camera fusion. SAE International Journal of Passenger Cars-Electronic and Electrical Systems, 10(1), 173-181.
Ulfarsson, G. F., & Shankar, V. N. (2003). Accident count model based on multiyear cross-sectional roadway data with serial correlation. Transportation Research Record, 1840(1), 193-197.
Virdi, N., Grzybowska, H., Waller, S. T., & Dixit, V. (2019). A safety assessment of mixed fleets with connected and autonomous vehicles using the surrogate safety assessment module. Accident Analysis & Prevention, 131, 95-111.
Wang, C., & Stamatiadis, N. (2014a). Derivation of a New Surrogate Measure of Crash Severity. Transportation research record, 2432(1), 37-45. https://doi.org/10.3141/2432-05
Wang, C., & Stamatiadis, N. (2014b). Evaluation of a simulation-based surrogate safety metric. Accident Analysis & Prevention, 71, 82-92. https://doi.org/https://doi.org/10.1016/j.aap.2014.05.004
Wang, C., Xu, C., & Dai, Y. (2019). A crash prediction method based on bivariate extreme value theory and video-based vehicle trajectory data. Accident Analysis & Prevention, 123, 365-373. https://doi.org/https://doi.org/10.1016/j.aap.2018.12.013
Wang, X., Xie, K., Abdel-Aty, M., Chen, X., & Tremont, P. J. (2014). Systematic approach to hazardous-intersection identification and countermeasure development. Journal of transportation engineering, 140(6), 04014022.
Washington, S., Haque, M. M., Oh, J., & Lee, D. (2014). Applying quantile regression for modeling equivalent property damage only crashes to identify accident blackspots. Accident Analysis & Prevention, 66, 136-146.
Wood, G. (2005). Confidence and prediction intervals for generalised linear accident models. Accident Analysis & Prevention, 37(2), 267-273.
Wu, J., Xu, H., & Zheng, J. (2017). Automatic background filtering and lane identification with roadside LiDAR data. 2017 IEEE 20th International Conference on Intelligent Transportation Systems (ITSC),
Wu, J., Xu, H., Zheng, Y., & Tian, Z. (2018). A novel method of vehicle-pedestrian near-crash identification with roadside LiDAR data. Accident Analysis & Prevention, 121, 238-249.
Wu, K.-F., & Jovanis, P. P. (2012). Crashes and crash-surrogate events: Exploratory modeling with naturalistic driving data. Accident Analysis & Prevention, 45, 507-516.
Wu, K.-F., & Jovanis, P. P. (2013). Defining and screening crash surrogate events using naturalistic driving data. Accident Analysis & Prevention, 61, 10-22. https://doi.org/https://doi.org/10.1016/j.aap.2012.10.004
Xie, K., Yang, D., Ozbay, K., & Yang, H. (2019). Use of real-world connected vehicle data in identifying high-risk locations based on a new surrogate safety measure. Accid Anal Prev, 125, 311-319. https://doi.org/10.1016/j.aap.2018.07.002
Ye, X., Pendyala, R. M., Shankar, V., & Konduri, K. C. (2013). A simultaneous equations model of crash frequency by severity level for freeway sections. Accident Analysis & Prevention, 57, 140-149.
Zhang, X., Liu, P., Chen, Y., Bai, L., & Wang, W. (2014). Modeling the frequency of opposing left-turn conflicts at signalized intersections using generalized linear regression models. Traffic injury prevention, 15(6), 645-651.
Zhao, M., Liu, C., Li, W., & Sharma, A. (2018). Multivariate Poisson-lognormal model for analysis of crashes on urban signalized intersections approach. Journal of Transportation Safety & Security, 10(3), 251-265.
Zheng, L., & Ismail, K. (2017). A generalized exponential link function to map a conflict indicator into severity index within safety continuum framework. Accident Analysis & Prevention, 102, 23-30. https://doi.org/https://doi.org/10.1016/j.aap.2017.02.013
Zheng, L., Ismail, K., & Meng, X. (2014). Freeway safety estimation using extreme value theory approaches: A comparative study. Accident Analysis & Prevention, 62, 32-41. https://doi.org/https://doi.org/10.1016/j.aap.2013.09.006
Zheng, L., Ismail, K., Sayed, T., & Fatema, T. (2018). Bivariate extreme value modeling for road safety estimation. Accident Analysis & Prevention, 120, 83-91. https://doi.org/https://doi.org/10.1016/j.aap.2018.08.004
Zheng, L., & Sayed, T. (2019a). Application of Extreme Value Theory for Before-After Road Safety Analysis. Transportation research record, 2673(4), 1001-1010. https://doi.org/10.1177/0361198119841555
Zheng, L., & Sayed, T. (2019b). Bayesian hierarchical modeling of traffic conflict extremes for crash estimation: A non-stationary peak over threshold approach. Analytic Methods in Accident Research, 24, 100106. https://doi.org/https://doi.org/10.1016/j.amar.2019.100106
Zheng, L., & Sayed, T. (2019c). Comparison of Traffic Conflict Indicators for Crash Estimation using Peak Over Threshold Approach. Transportation research record, 2673(5), 493-502. https://doi.org/10.1177/0361198119841556
Zheng, L., & Sayed, T. (2019d). From univariate to bivariate extreme value models: Approaches to integrate traffic conflict indicators for crash estimation. Transportation Research Part C: Emerging Technologies, 103, 211-225. https://doi.org/https://doi.org/10.1016/j.trc.2019.04.015
Zheng, L., & Sayed, T. (2020). A bivariate Bayesian hierarchical extreme value model for traffic conflict-based crash estimation. Analytic Methods in Accident Research, 25, 100111. https://doi.org/https://doi.org/10.1016/j.amar.2020.100111
Zheng, L., Sayed, T., & Mannering, F. (2021). Modeling traffic conflicts for use in road safety analysis: A review of analytic methods and future directions. Analytic methods in accident research, 29, 100142.
Zhou, S., Sun, J., An, X., & Li, K. (2011). The development of a conflict hazardous assessment model for evaluating urban intersection safety. Transport, 26(2), 216-223.
Żywanowski, K., Banaszczyk, A., & Nowicki, M. R. (2020). Comparison of camera-based and 3d lidar-based place recognition across weather conditions. 2020 16th International Conference on Control, Automation, Robotics and Vision (ICARCV),